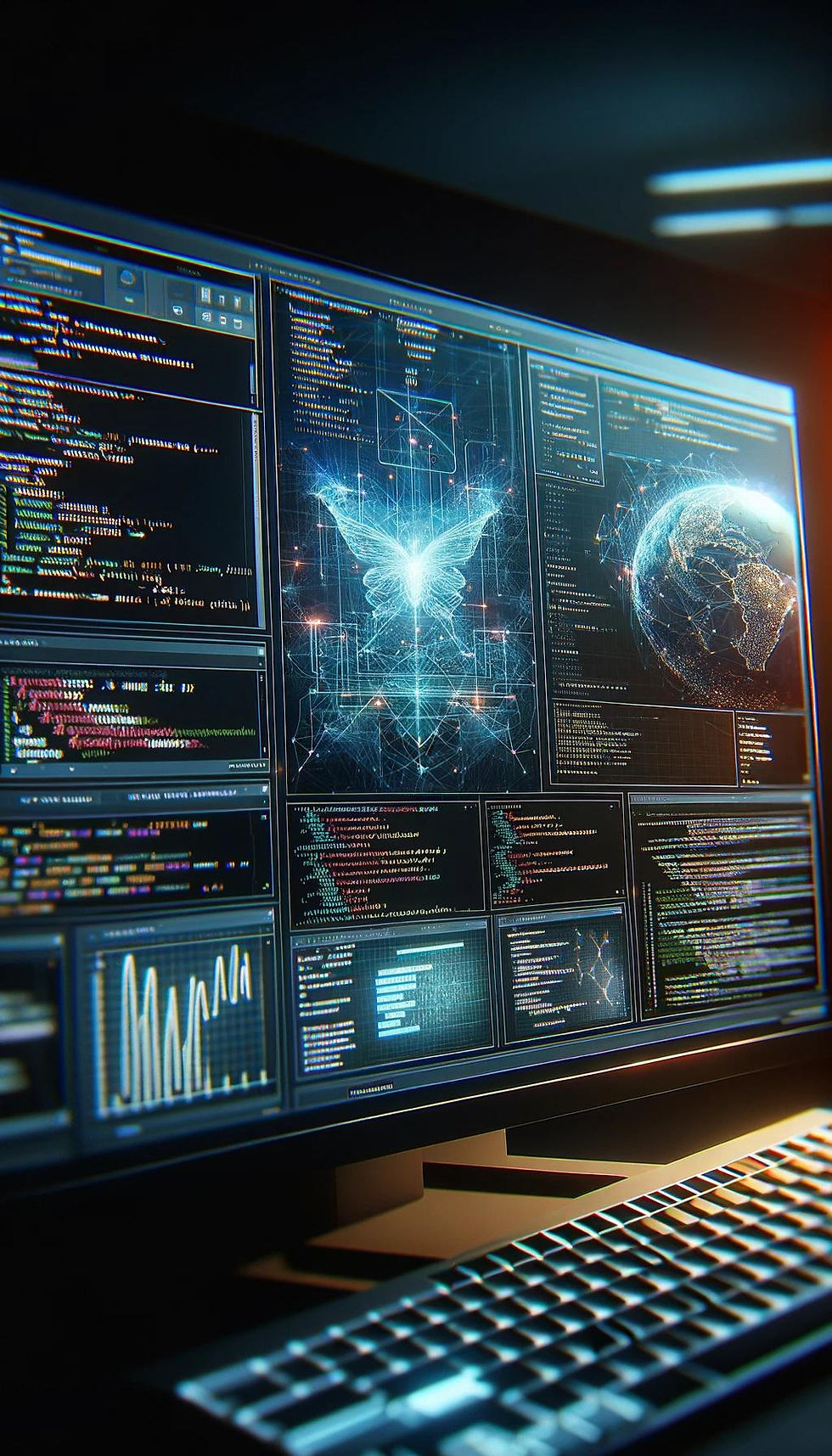
Hey there! So, we all know that artificial intelligence (AI) has the potential to totally shake up our world, from automating tasks to making experiences more personalized. But, there is a sneaky issue lurking in the background – AI bias. Just like humans, AI algorithms can pick up and magnify societal biases, which can lead to unfair outcomes.
Picture this: an AI-powered system for approving loans that ends up favoring applicants from specific zip codes, basically keeping old housing inequalities alive. Or, a facial recognition system that doesn't work as well for people of color, potentially affecting decisions in the justice system. These aren't just made-up scenarios; they're real examples of AI bias with serious consequences.
But here's the good part: data science, the very thing powering AI, can also be our way to fight bias. By understanding how bias creeps into AI systems and using data science techniques, we can make AI that's fairer and more ethical for the future.
The Sources of AI Bias:
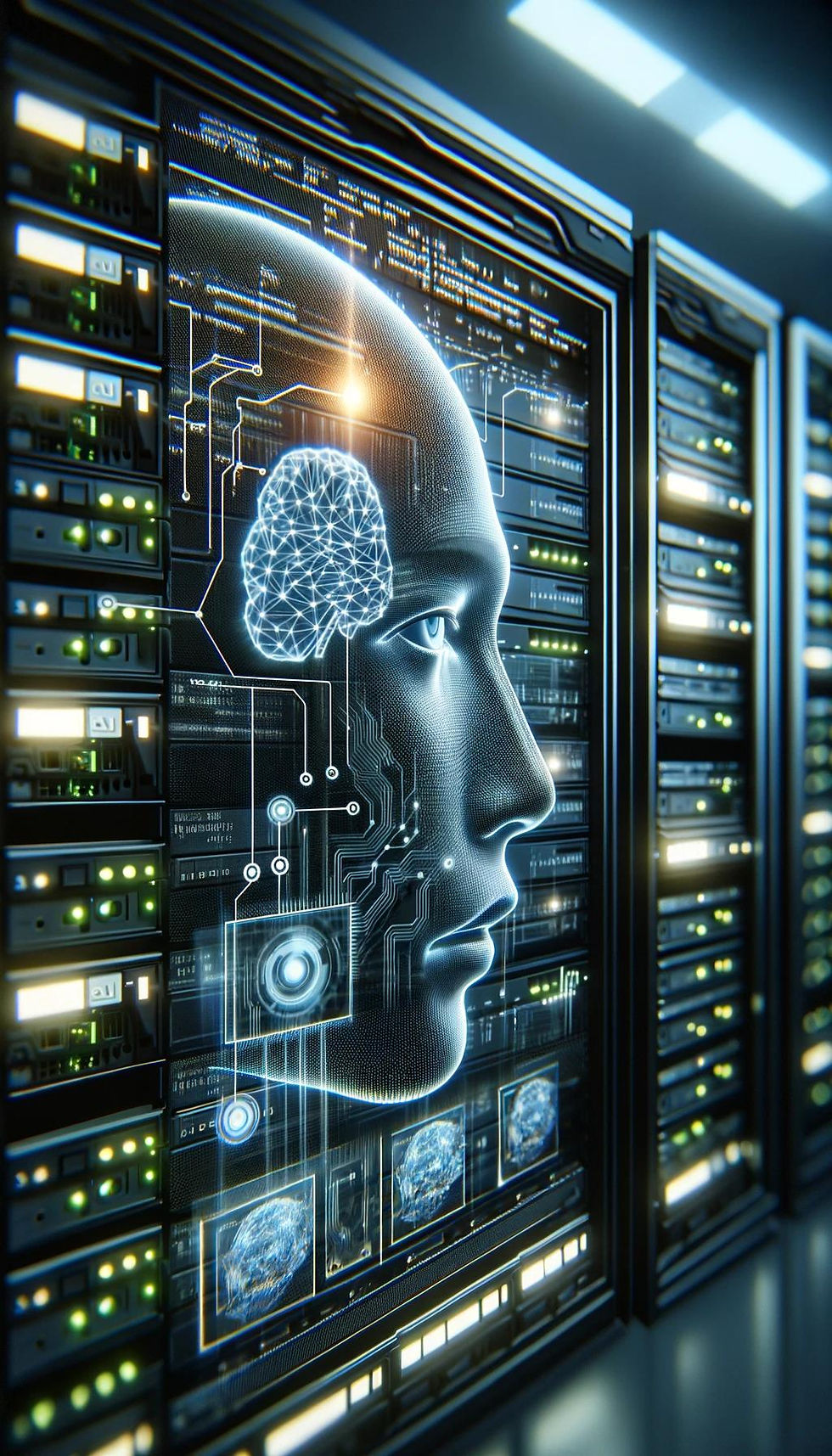
There are three main reasons why AI can be biased:
Biased Data: AI learns from data, so if the info it's trained on reflects societal prejudices, the AI model will pick up those biases. For example, a resume screening AI trained on data biased against female applicants might end up perpetuating that bias in its own decision-making.
Algorithmic Bias: The algorithms themselves can introduce bias. For instance, some algorithms might focus more on accuracy for the majority group, which means they end up with less accurate results for minority groups.
Human Bias: The people developing and designing AI systems might unknowingly add in their own biases through the choices they make and the metrics they use.
How Data Science Can Help Reduce Bias:
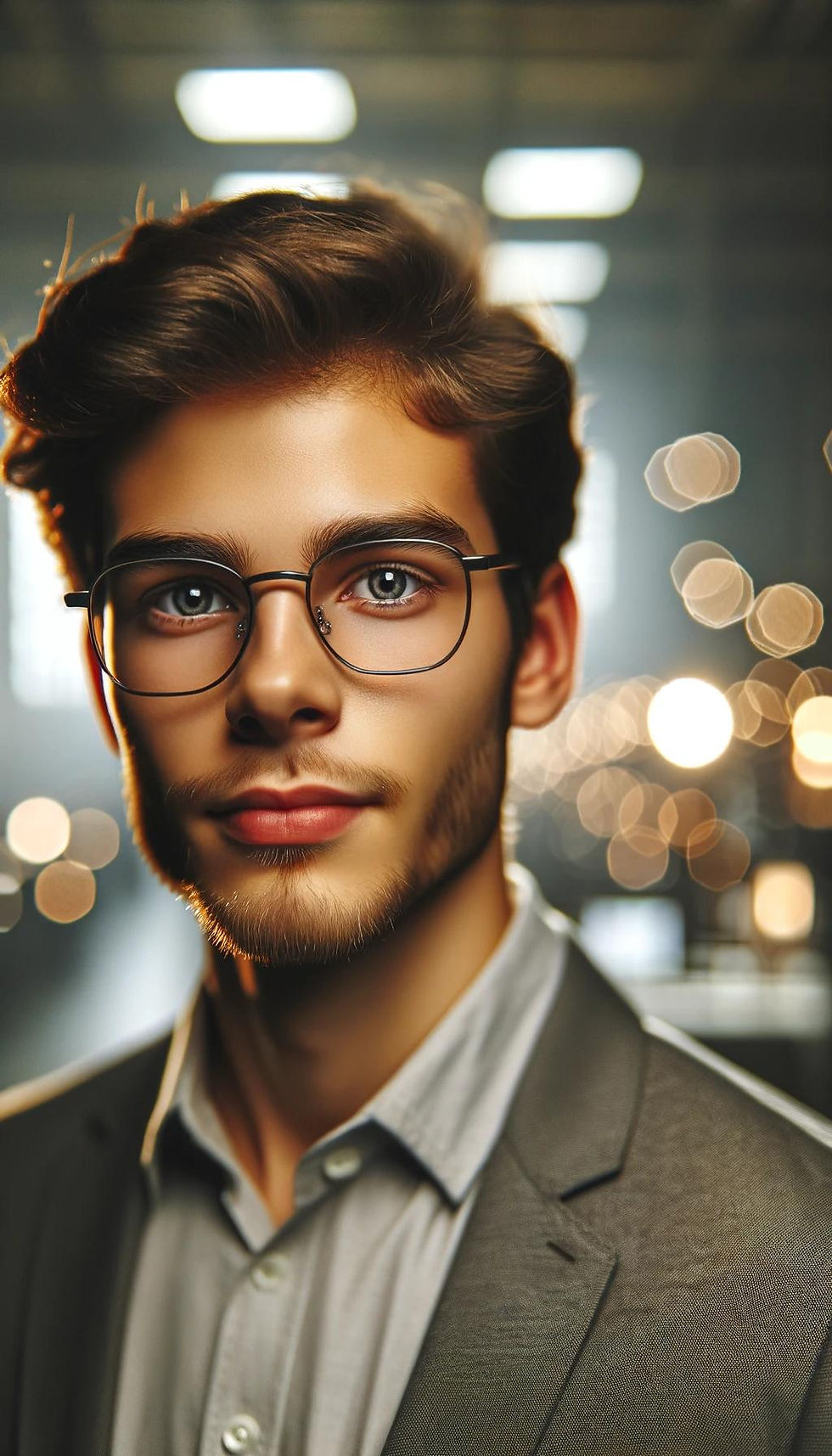
Data scientists are the ones who can really make a difference in tackling AI bias. Here are a few ways they can do that:
Data Cleaning and Augmentation: Techniques like cleaning biased entries from data and creating more diverse datasets can really improve fairness. Just imagine adding extra factors like education and work experience to a loan approval dataset, so it's not so dependent on zip codes.
Fairness Metrics and Algorithm Choices: Data scientists can use fairness metrics to spot bias in algorithms and think about using different algorithms that focus on fairness as well as accuracy.
Explainable AI (XAI): Making AI models that are clear and easy to understand lets humans see how AI makes decisions, which can reveal any hidden biases.
Real Examples of Fighting Bias:
Here are a few examples of how data science is being used to deal with AI bias:
Amazon's Rekognition facial recognition software: It got called out for racial bias at first, but Amazon used data science techniques to make it more accurate across different groups of people.
Apple Card's creditworthiness algorithm: Accused of gender bias, Apple looked at its model again using fairer data and making algorithm changes.
Project Debias: This is a Microsoft initiative that uses fairness toolkits to help developers spot and reduce bias in their AI systems.
Looking Ahead:
The battle against AI bias is ongoing, but here's what we can take away for the future:
Diversity in AI Teams: Having diverse teams working on AI can bring in different perspectives and help spot biases early on.
Transparency and Explainability: Making AI models that are clear and easy to understand is really important for building trust and making sure AI is used ethically.
Regulation and Standards: Having ethical guidelines and rules for AI development can help reduce bias and push for responsible innovation.
Comentarios